Vectara Announces Partnership with LangFlow
Vectara and LangFlow announce integration of Vectara state-of-the-art Retrieval Augmented Generation and chat service with LangFlow’s easy-to-use no-code engine
Building an enterprise-scale RAG application requires dealing with lots of complex details: chunking, embedding, advanced retrieval (like hybrid search or MMR), and using the LLM correctly for summarization. When applied at scale, this is more complicated than it initially appears.
"At Vectara, we understand the complexity of building enterprise RAG that is scalable and secure, providing easy adoption by enterprise IT teams," said Amin Ahmad, co-founder and CTO of Vectara. "All of the work we put into building the serverless RAG infrastructure behind Vectara’s platform is now available immediately to all users of LangFlow.”
LangFlow has a vibrant community of users that utilize a wide array of components from the Langflow Store to build GenAI applications. With this partnership, LangFlow users can avoid the endless experimentation involved in stitching various data loaders, LLMs, and prompts together, and instead gain direct access to Vectara’s end-to-end RAG flow for question-answering, chatbots, or semantic search.
“We are thrilled to add this integration with Vectara. RAG continues to be adopted in the enterprise and the Vectara component significantly simplifies the effort needed to build Retrieval applications and reduces time-to-value,” said Rodrigo Nader, CEO and co-founder of LangFlow.
The GenAI market remains evolving at a rapid speed, and applications such as chatbots, questions answering, and research continue to enhance productivity across legal, healthcare, insurance and many other industry verticals.
“The combination of no-code from LangFlow with the RAG-in-a-box solution from Vectara helps practitioners quickly explore the applications that are most valuable to them,” said Ofer Mendelevitch, Head of Developer Relations at Vectara. “Users can be confident that their solution based on Vectara scales to meet their enterprise needs, and provide CISOs and CIOs the peace of mind they need to deploy GenAI applications in their organizations.”
About Vectara
Vectara is an end-to-end platform for embedding powerful generative AI features into applications with extraordinary results. As an end-to-end Retrieval Augmented Generation (RAG) service, Vectara delivers the shortest path to a correct answer/action through a safe, secure, and trusted entry point. Vectara never trains on your data, allowing businesses to embed generative AI capabilities without the risk of data or privacy violations. To learn more about Vectara, visit www.vectara.com.
About LangFlow
LangFlow is an intuitive and versatile no-code AI platform tailored for seamless integration into existing technology stacks, offering a straightforward approach to leveraging the full potential of Generative AI. Designed to bridge the gap between complex AI development and practical, real-world application, LangFlow provides a Python-native ecosystem that simplifies the creation and sharing of autonomous agents, retrieval pipelines, and much more. With a focus on enabling fine-grained control over multiple sequential calls to language models, LangFlow facilitates a chat-like or workflow pipeline that serves a diverse range of AI prototyping and deployment scenarios. By offering pre-built components that can be combined in countless configurations, it stands out as the simplest and smartest pathway to developing around Foundation Models, empowering users to build, experiment, and deploy AI applications with unprecedented ease and flexibility. To explore the possibilities of LangFlow and start building your AI-driven solutions today, visit www.langflow.org.
Carly Bourne
Vectara
+1 423-443-0449
email us here
Visit us on social media:
Facebook
Twitter
LinkedIn
Instagram
YouTube
Other
WATCH NOW: Vectara and LangFlow Tutorial: Building a RAG Chatbot! at https://www.youtube.com/watch?v=VP0NDf2C3Kg
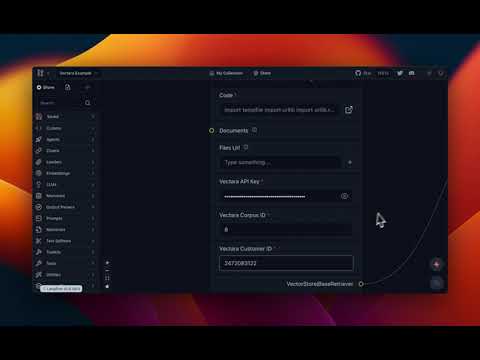
1 https://vectara.com/
2 https://www.langflow.org/
3 https://www.langflow.store/store
